Why ChatGPT technology will change arable farming
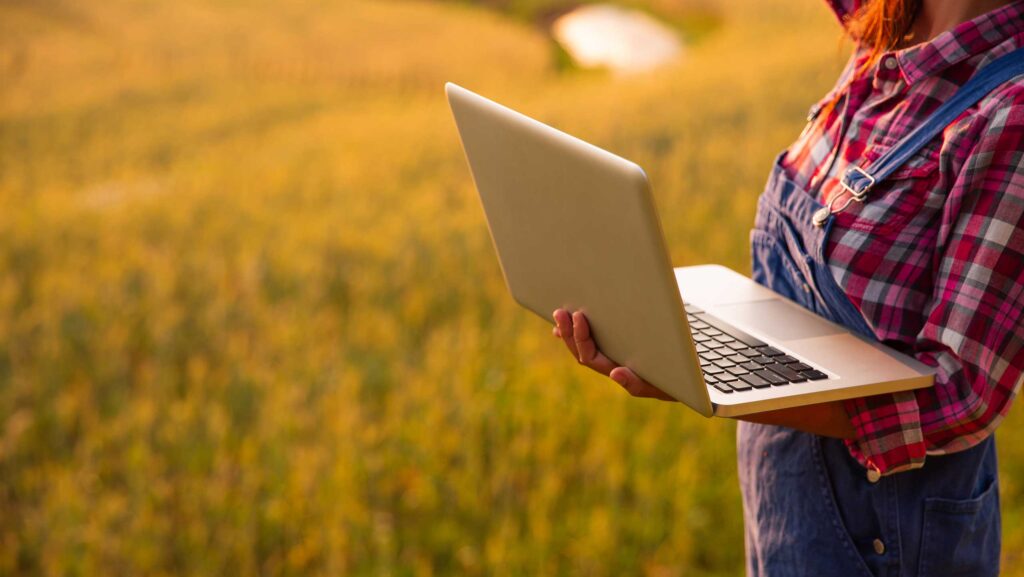
Co-pilots for agronomists and farmers, rapid advances in seed breeding, and the end to colourful but relatively useless yield maps are just three of the initial applications for what is being hailed as the next disruptive technology in agriculture.
While the term generative artificial intelligence (gen AI) may not be that familiar yet, it’s the technology behind the chat-based interface ChatGPT. This tech attracted more than 100 million users worldwide in just two months after its launch in November 2022.
The key difference between artificial intelligence and the new sub-branch of gen AI is that while AI was used to understand and analyse data, gen AI created new content, explained Dr Elliott Grant, chief executive of the AI company Mineral, who was speaking at the World Agritech Innovations Summit in San Francisco earlier this year.
See also: Blackgrass control as later-germinating populations emerge
For example, while self-driving cars and plant perception technology use AI, converting text to video or images uses gen AI.
Within agriculture, the first disruptive impact of gen AI applications was likely to be the way humans interacted with data, Elliott suggested.
“That will be huge: no more multi-coloured maps or wildly over-complicated dashboards or spreadsheets. We can now ask a natural question of our data, and gen AI will take the question, decompose it into a query, run analysis and generate a summary back to the user.
“The way we will interact with data over the next few years will be radically different.”
Microsoft’s chief technical officer of agri-food, Ranveer Chandra, agreed. “There are two key things that generative AI enables,” he told the conference.
“First, it changes the way we interact with computers – you don’t need a mouse or keyboard. You can talk to the app and it can parse huge amounts of data and come back with an answer.
“And second, it is an intelligence engine. You give it data and it can come up with insights on top of that data.”
Virtual agronomist chatbot
The potential for AI means every major player in agriculture is experimenting with gen AI, but one of the first areas to gain some traction is a virtual agronomist chatbot – an AI agronomist.
Global non-profit Digital Green began rolling out its AI assistant to agronomists in India, Kenya and Ethiopia in 2023. In some areas, there may be only one agronomist for up to 1,000 farmers, making it much cheaper and faster to use a chatbot than to send an agronomist to a remote area.
Initially, however, Digital Green is targeting its chatbot, which is based on Telegram, a messaging service similar to WhatsApp, to help those agronomists quickly research and answer farmer questions, saving critical time in providing the timely, accurate, localised advice the farmers need and thereby increasing farmer faith in their advice.
Building agronomist co-pilots is happening elsewhere. For example, Bayer launched a gen AI agronomist assistant earlier this year at the World AgriTech Innovation Summit in San Francisco, while Syngenta also teased something similar at the event.
Bayer’s version uses proprietary agronomic data, plus other publicly available data, to train a large language model.
The result from the internal data, including insights from trials, and aggregated experience from its agronomists, is an expert system that quickly answers questions about agronomy, farm management and relevant Bayer products.
Again, like the Digital Green product, the firm claims the technology gives the adviser the information required to answer grower questions, and helps give higher quality recommendations.
Turn data into insights
The system was initially piloted with Bayer teams in the US, and the firm aims to expand that to selected advisers and potentially farmers this year.
It is also developing a separate gen AI prototype, in partnership with Microsoft, that will allow farmers to directly query their own farm data.
“We’re looking at how we can leverage this technology to reduce the friction for advisers and farmers to turn data into insights,” explained Dr Jeremy Williams, head of digital farming at Bayer.
“It will allow farmers to interact with their data in a more natural way. For example, asking things like which fields had over a certain amount of rainfall, or what was the difference between my expected yield and what I harvested.”
One of the keys to whether this and other gen AI systems would ultimately be widely adopted was value, said Nalini Polavarapu, Bayer vice-president of enterprise analytics and data science.
“The value has to be clear,” she said. “Farmers are very practical; their margins are small, and they don’t have many shots, so when AI is providing recommendations it has to be accurate.
“If Netflix or Amazon makes a recommendation for something to watch, and the person watching doesn’t like it, they might curse you for wasting a couple of hours, but it’s not bad.
“In farming, it can really impact your livelihood, so it is important AI models provide value, and for that we need very high-quality data.”
And that’s where some of the challenges in developing accurate models currently lie – both in terms of the granularity of data required and in allowing diverse datasets to be merged or aggregated.
“If we can tackle that, it will help solve a lot of the problems with adoption,” Nalini predicted.
Industry analyst Shane Thomas believes tools that help agronomists manage tedious administrative functions, rather than agronomy decision-making, are likely to be adopted, at least initially.
Writing in his weekly Substack newsletter, Upstream Ag Insights, Shane says agronomic decision-making, as with farm management software, is a difficult space to win in.
“Does a farmer or agronomist want an agronomic question answered by a crop protection company model, or would they prefer it from an independent or less biased entity?” he asks.
Another issue is whether farmers and agronomists would want to use multiple standalone models, especially if they only relate to agronomy.
“Models are better off starting with transactional and admin tasks of agribusiness professionals,” he suggests.
“These not only alleviate more day-to-day pain points all year round, but the stakes are also lower, meaning if the inventory of a product comes back incorrect, it’s minor versus an incorrect agronomic suggestion.”
AI agronomic assistant
US tech firm Headstorm has followed that logic in building its AI agronomic assistant, Agpilot, for US distributor adviser teams. It provides quick access to customer overviews, including crop types and areas, yields and costs per acre, sales records and inventories, through a chatbot-type interface.
The agronomist also receives proactive notifications of weather events and pest or disease outbreaks, which the app can also turn into draft customised messages for affected growers, increasing adviser productivity and freeing time to manage more customers.
Building trust in a model’s insights and outputs is another key challenge for gen AI developers to achieve.
Anyone who has used ChatGPT or similar will probably have come across outputs that are incorrect – so called hallucinations.
That was an incredibly important topic, acknowledged Elliott. “The [AI] industry has matured to a point where it’s not a gimmick anymore, so we have an obligation to farmers and the industry that they can trust it. But there is a gap to close before we get there.”
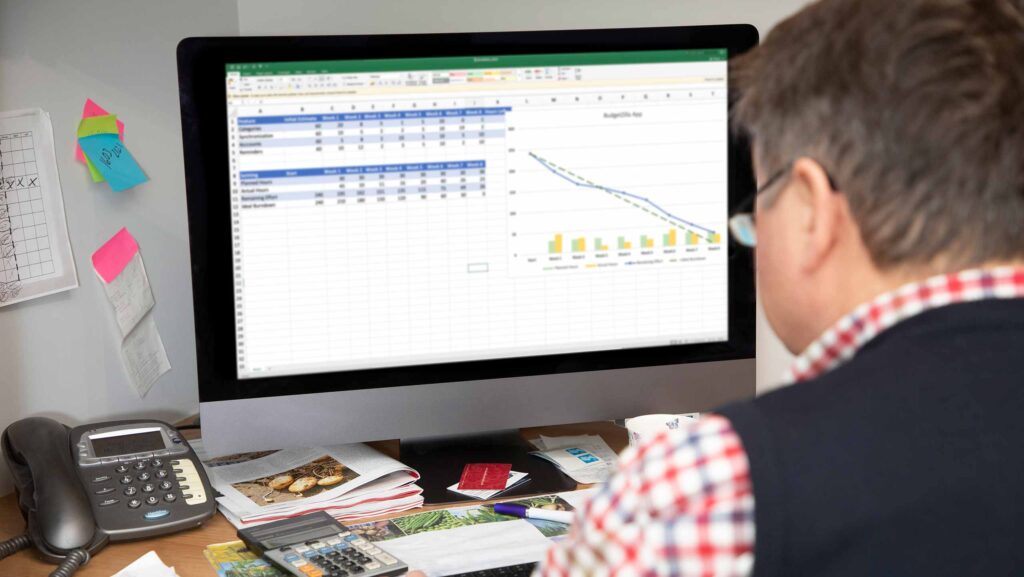
© GNP
Definitions: How do AI, machine learning, deep learning and gen AI fit together?
- Artificial intelligence (AI) is the science and engineering of making intelligent machines that can replicate human behaviour, including tasks related to perceiving, reasoning, learning and problem-solving.
- Machine learning is a major breakthrough in achieving AI. Algorithms detect patterns in large data sets and learn to make predictions by processing data, rather than by receiving explicit programming instructions.
- Deep learning is an advanced type of machine learning, using neural networks, inspired by the human brain, to ingest data and process it through multiple iterations that learn increasing complex features and make more sophisticated predictions.
- Generative AI (gen AI) is an advanced branch of deep learning that uses exceptionally large neural networks, called large language models, that can learn especially abstract patterns. Language models applied to interpret and create text, video, images and data are known as generative AI.
Source: McKinsey
To build that trust, gen AI developers in agriculture needed to work together to build similar, independently validated golden datasets – as were being used in healthcare – that new technologies could be tested against, he suggested.
“If someone wants to come up with a new AI model for brain tumour detection, it must be tested against this third-party dataset to validate its claims,” he explained. “In agriculture, we don’t have that, and I think we desperately need it.”
Developers being able to explain a model’s outputs was also crucial to building trust, he said.
“We have an obligation for certain applications to explain why the model is doing what it is doing, so that means chain-of-thought reasoning.
“So what’s the model paying attention to?”
Elliott said there was also an obvious need to avoid bias in the datasets.
“If we train our models on corn and soybeans grown in the US Midwest, it’s not going to wake up one day and discover regenerative agriculture or smallholder cassava farming.
“We have to very intentionally train on balanced data and to have non-conventionally grown commercial crops in our datasets.
“And if we want that type of future, [tech developers and others in the industry] have to do it together.”
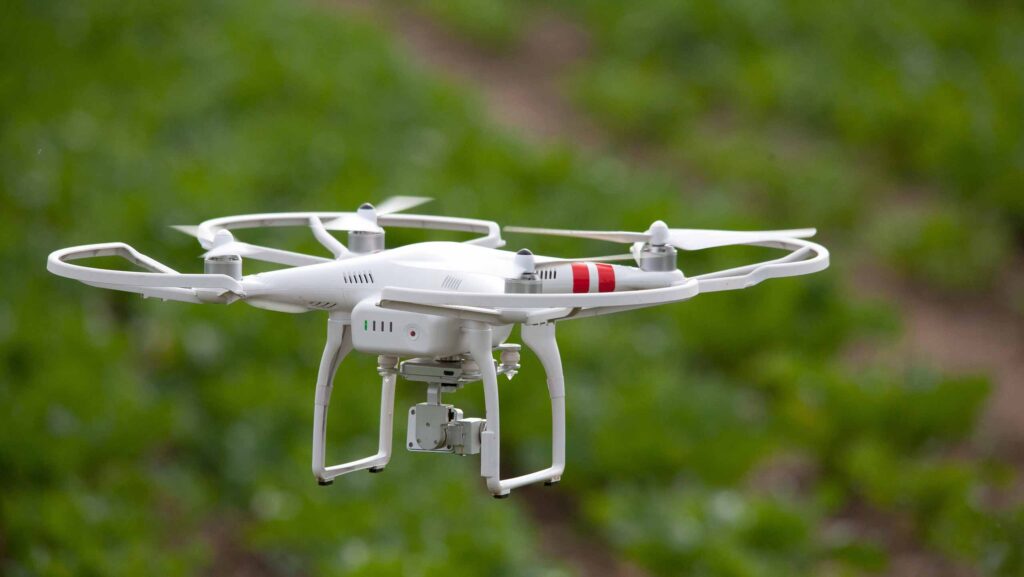
© Blackthorn Arable
Farmers’ trust
Developers within ag businesses also needed to be very clear on the way they were leveraging farmer data, Jeremy added.
“We have to be confident that we are using it in ways they permit.”
But despite the challenges around trust, data and creating value for farmers, there is a consensus that both use and types of gen AI models will grow exponentially in the next five years.
Predicting the future direction of the tech was virtually impossible, given how quickly it was changing, Elliott said.
“But I think there is the opportunity for a paradigm shift in agriculture, enabled by AI and gen AI, to a mode of continuous model retraining, which unlocks the ability to do continuous monitoring and management.
“We’ll no longer have farm equipment that goes into the field once or twice a season, but farm equipment that is in the field continuously, monitoring and learning.”
That would radically change a farmer’s risk calculation, trading horsepower for brainpower of the AI type, he suggested, reducing exposure to single, expensive machines in favour of software-defined, low-cost machines stacking dozens of smaller, lower risk decisions through the season rather than a few high-stakes ones.
Predictions for gen AI breakthroughs and outcomes
- A large language model that understands plant genomes and can predict changes in plant characteristics and traits from changes in the genetic code
- Faster innovation including products coupled with precision applications which would not otherwise have made it through pipelines
- Training foundational image models with synthetic images generated by gen AI, reducing time and expense to produce models that accurately detect specific pests, weeds or diseases
Case study: UK grower finds value in gen AI applications
East Anglian arable farmer James Sills is already getting value from his business subscriptions to Open AI’s premium version of ChatGPT and Anthropic’s Claude 3 Opus chatbots
“It’s an entirely legitimate business expense,” he says.
The subscriptions cost him about £20/month, and he finds the conversational AI bots extremely useful for tasks where he doesn’t need 100% accuracy immediately.
“It’s very useful for making first drafts. For example, we used it for a job advert. Instead of taking me 45 minutes to do a first draft, it can do it in two minutes.
“I also often use it to summarise long documents. Recently I wanted to read something on urea’s effect on wheat. It was 15 pages long and I had five minutes.
“Instead of reading it all, I asked what the key lessons from this document are and where do I find them in the document, and in five minutes I could do what would have taken 35 minutes previously.”
That doesn’t always work, he admits. “I used it to summarise Niab strategy documents and it didn’t do a very good job.”
Other tasks where it has helped include understanding key pieces of employment law and checking insulation values for rental houses.
It’s also been useful for machinery repairs. “I needed to swap the wires in an electrical connector, so I took a picture and asked how I did it and got the answer pretty quickly. Beforehand I didn’t even know it was called a Deutsche connector, so I wouldn’t have known what words to use to search.”
There are some caveats, he stresses. “I can point to where it’s definitely been useful, but if I’d followed it blindly, it would have lost me a lot of money.”
He used it to work out what diameter of cable he needed to use from his main electricity supply to a solar panel installation. It had used the right equations and looked impressive.
But subsequently, he discovered it was out by a factor of two. “If we’d followed its recommendation, we would have got ourselves in a mess” he admits.
“It’s the hallucination problem, which seems to be the major hurdle currently.”
James says the range of possible future uses seems vast as the technology develops. “I think the prospects are difficult to get your head around, but even if gets no better than it is now, I think it is disruptive technology, particularly when you consider how much using voice and images could make it easy to use.”
In July, Mineral announced it would no longer be an Alphabet company, with some of its technology being transferred to berry grower Driscoll’s, which it had been partnering with.