3 adoption steps for AI to transform arable farming
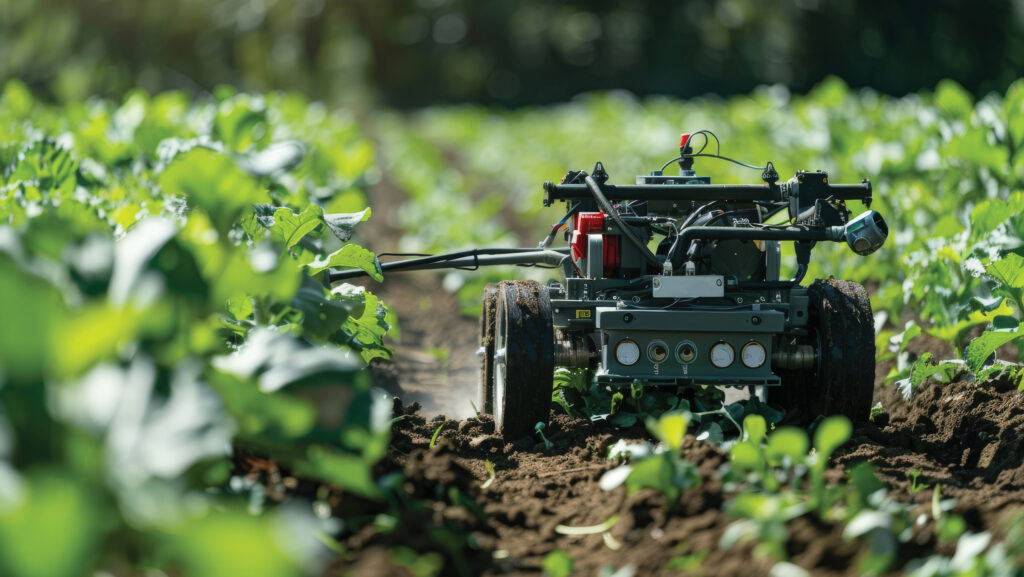
Technologies using artificial intelligence (AI) are primed to progressively change the way we farm, ultimately leading to a fundamental shift in the way farmers grow crops.
That’s the prediction of Dr Elliott Grant, who spent eight years leading Google’s work on AI within agriculture, as chief executive officer of Mineral.
There will be three phases for how AI will integrate into agriculture, he suggests.
See also: How 3D crop mapping can future-proof potatoes
Substitution
Initial adoption of AI within agriculture will be using it to substitute boring or repetitive tasks farmers currently perform.
Tedious, often digitally based jobs, are prime examples of the “low-hanging fruit” for AI, as they can easily be automated through algorithms and rule-based systems, Elliott explains.
Examples include filling in forms, summarising information and writing basic emails.
But substitution will extend further than just office-based tasks.
For example, Mineral have developed phenotyping robots using computer vision and machine learning algorithms that measure rust in wheat crops five times faster than experts in a side-by-side comparison by the University of Minnesota.
“The robot can do what a human does, equally as well, and more cheaply and reliably,” Elliott says.
Augmentation
Adoption of AI will quickly extend to tools that can enhance human capabilities by providing insights or doing activities that weren’t previously economical.
For example, unlike human researchers, the phenotyping robot won’t get tired or bored collecting rust data, and could be sent out every day to collect information.
Elliott suggests: “It’s also difficult for human expertise to be consistent.
“Robots are super consistent, and you could have a robot in five different locations at the same time measuring wheat rust.”
That’s an improvement on the current method of assessing disease levels, making it more comprehensive, he says. On-farm, this could enable earlier intervention, lowering costs and reducing crop losses.
A second example is AI-powered sprayers which can precisely target individual weeds while travelling at production speeds of 15mph, potentially reducing herbicide use by up to 90%.
“This is an augmentation as farmers broadcast spray,” Elliott says.
“The opportunity for a machine like this is to dramatically reduce the cost to the farmer of the herbicides.
“The economics are very compelling, and the industry is pushing down the cost curve to make this readily available to farmers.”
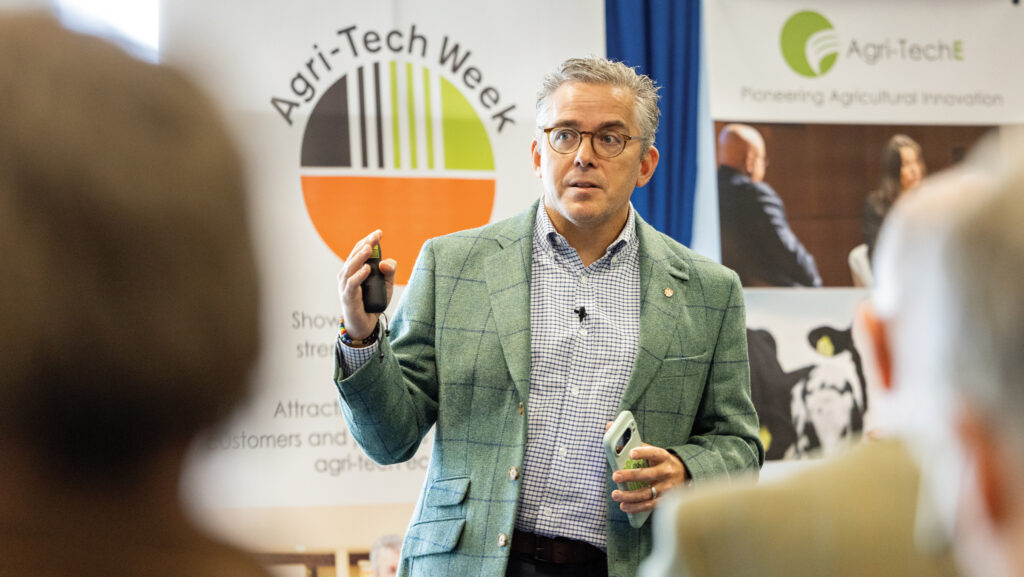
Elliott Grant © AgriTechE
Transformation
The final stage of AI’s impact will likely be powering a fundamental shift in agricultural practices and business models, Elliott suggests.
“This is further away, but one I think 10 years from now we’ll be looking back and think we didn’t see that coming.
“It will be where we don’t work the same way as today and do jobs completely differently.
“I can’t tell you what it is going to look like, but it won’t just be an automated version of today. It’s potentially something radically different and hopefully better, more sustainable, profitable for farmers and better for the planet.”
An example of a potential transformation he envisions is a move from today’s “episodic” crop management (using large, heavy machinery) to “continuous” crop management.
That might build on the spot-spraying technology, but with a fleet of perhaps slower-moving, lighter, more agile machines that are in fields continuously monitoring and managing crops at an individual plant level, he suggests.
“It seems crazy to imagine every single crop plant in a field being managed perfectly at a per-plant level, but I do think that’s going to happen in the next 10-20 years.”
Challenges
While the use of AI is inevitable, and Elliott is optimistic about its potential, he stresses there are challenges that must be addressed.
“We have an obligation as developers, technologists and as government regulators to mitigate unintended consequences and negative effects.”
Ethical considerations – for example, avoiding bias when training models – will be crucial, while data privacy is another thorny issue.
Striking a balance between data privacy concerns and the need for comprehensive datasets to train effective AI models is vital.
Farming data is extremely valuable, Elliott recognises.
“It’s often your differentiator and you can’t give it away for free. On the other hand, if you don’t share data then we never get the benefit of common learning.
“There’s a paradox that if you keep data to yourself, you get to be different, but you’ll never get that much better.
“I think the answer to that paradox is to find a way to share data in a way that doesn’t disclose private information.”
That is already happening in the healthcare sector, where a mechanism known as confidential computing is used to allow models to learn using shared private data, without anyone’s data leaking, he says.
Another challenge is around trust. Mineral already developed a model that was better than humans at predicting strawberry yields two weeks into the future.
“But understanding why is hard because AI tends to be a black box – you don’t know what it is doing.”
While in this case they worked out that it had identified certain attributes that human experts weren’t taking into account, the way AI works by iterating billions of times and writing new code means, in most cases, understanding what it is doing will be unknowable, he explains.
“It’s like having the world’s best agronomist who just can’t explain themselves,” Elliott says.
“Do you want that agronomist? It’s a double-edged sword, because we could potentially have the most powerful insightful agronomy expertise, but we won’t understand why anymore.”
Business model innovation will be key: “We need to think about business models that make sure the value flows back to the farmer.
“It could be a retailer buying differently, a wholesaler having a different contract, different financing of farm equipment, or a risk-sharing agreement.
“All these need to be considered because the way we do it today isn’t going to work,” he concludes.
Information for this article was gathered at the 2024 Agri-TechE REAP Conference
New market could produce coffee alternative
A start-up is looking to use UK-grown crops such as barley and pulses to produce a coffee alternative.
As well as being the world’s favourite drink – with 3bn cups consumed globally a day – coffee has one of the most agriculturally and socially exploitative value chains, according to Anna-Sophie Deetjen, chief executive officer and co-founder of Morrow.
“The coffee in your cappuccino emits up to 17 times more carbon equivalent emissions than milk, uses enough water to fill two bathtubs and is a significant driver of deforestation, with over 2m hectares of wild forests replaced by coffee plantations between 2001 and 2015,” she says.
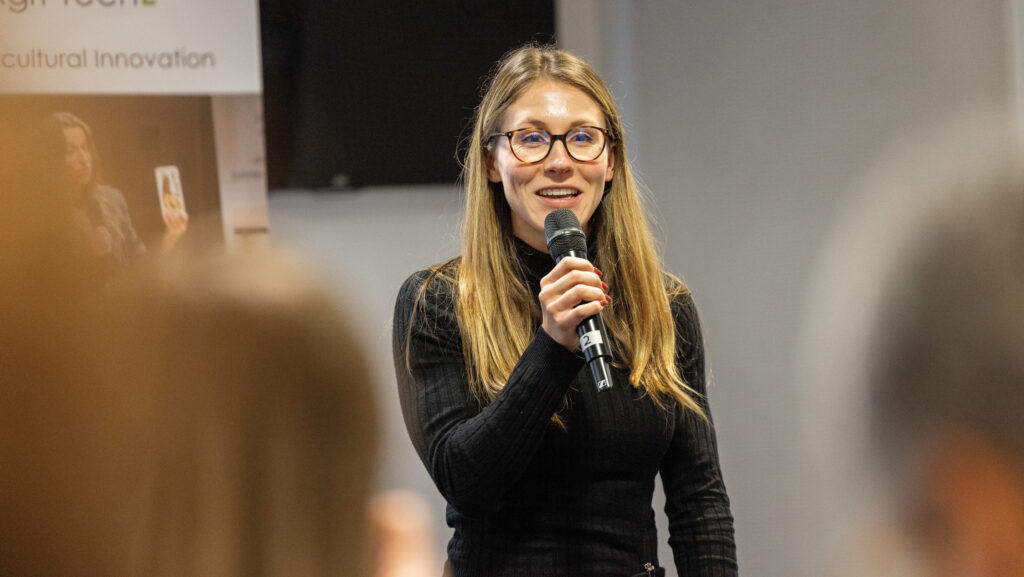
Anna-Sophie Deetjen © AgriTechE
In addition, there are predictions that climate change could mean 60% of land currently used to grow coffee might be unsuitable for production by 2050, which coupled with growing demand, could create a supply crisis.
“With this deficit in supply looming, we think there is a huge opportunity in the UK. We currently import over 150,000t of coffee worth £1.1bn every single year.”
Morrow’s plan is to fill that gap using a product created from pulses and grain crops grown in the UK, plus upcycled ingredients such as fruit pips and peels, Anna-Sophie says.
“Our product smells and tastes like coffee, but with a fraction of the carbon footprint – no deforestation while supporting local agricultural systems.”
Coffee flavour is determined by a combination of genetic and environmental factors, with components such as sugars, lipids and amino acids in the coffee bean acting as flavour precursors, which when roasted, come to life to form the coffee flavour, Anna-Sophie explains.
“Through advanced flavour chemistry profiling, we’re able to replicate some of these flavour notes using a number of biotransformations such as malting and roasting, essentially layering ingredients on top of each other to create a coffee flavour profile.
“We use data analysis and feedback from consumers, coffee experts and flavourists to fine tune our prototypes, which we are now scaling up for production and launch in 2025.”